Statistical Modelling And Inference Using Likelihood: A Comprehensive Guide

Statistical modelling is the process of using data to create a model that can be used to make predictions or inferences about the population from which the data was drawn. Inference is the process of using the model to make s about the population. Likelihood is a measure of how well a model fits the data. It is used in both model fitting and inference.
Likelihood Functions
A likelihood function is a function that measures the probability of observing the data given the model. It is often written as:
4.6 out of 5
Language | : | English |
File size | : | 31501 KB |
Text-to-Speech | : | Enabled |
Screen Reader | : | Supported |
Enhanced typesetting | : | Enabled |
Print length | : | 543 pages |
Lending | : | Enabled |
$$L(\theta | x) = P(x | \theta)$$
where:
* \(L(\theta | x)\) is the likelihood function * \(\theta\) is the model parameter * \(x\) is the data
The likelihood function is often used to estimate the model parameters. The maximum likelihood estimator (MLE) is the value of the model parameter that maximizes the likelihood function. The MLE can be found by solving the following equation:
$$\frac{\partial L(\theta | x)}{\partial \theta}= 0$$
The MLE is a point estimate of the model parameter. It is often used to make inferences about the population from which the data was drawn.
Hypothesis Testing
Hypothesis testing is the process of using data to test a hypothesis about the population from which the data was drawn. The hypothesis is typically a statement about the value of the model parameter. The null hypothesis is the hypothesis that the model parameter is equal to a specified value. The alternative hypothesis is the hypothesis that the model parameter is not equal to the specified value.
To test a hypothesis, we first calculate the likelihood ratio:
$$LR = \frac{L(\theta_0 | x)}{L(\theta_1 | x)}$$
where:
* \(LR\) is the likelihood ratio * \(\theta_0\) is the null hypothesis parameter value * \(\theta_1\) is the alternative hypothesis parameter value
The likelihood ratio is a measure of how much more likely the data is under the null hypothesis than under the alternative hypothesis. A large likelihood ratio means that the data is much more likely under the null hypothesis than under the alternative hypothesis. A small likelihood ratio means that the data is about as likely under the null hypothesis as it is under the alternative hypothesis.
We can use the likelihood ratio to test the hypothesis using a chi-squared test. The chi-squared statistic is:
$$\chi^2 = -2\ln(LR)$$
The chi-squared statistic is distributed as a chi-squared distribution with degrees of freedom equal to the difference between the number of parameters in the null and alternative hypotheses. We can use the chi-squared distribution to calculate the p-value for the hypothesis test. The p-value is the probability of observing a chi-squared statistic as large as or larger than the one we calculated. A small p-value means that the data is very unlikely under the null hypothesis. A large p-value means that the data is fairly likely under the null hypothesis.
If the p-value is less than the pre-specified significance level, we reject the null hypothesis. If the p-value is greater than the pre-specified significance level, we fail to reject the null hypothesis.
Model Selection
Model selection is the process of choosing the best model from a set of candidate models. The best model is the model that best fits the data and has the fewest parameters. There are a number of different criteria that can be used to select the best model, including:
*
Akaike Information Criterion (AIC): The AIC is a measure of the goodness of fit of a model. It is calculated as:
$$\text{AIC}= -2\ln(L(\hat{\theta}| x)) + 2k$$
where: * \(\hat{\theta}\) is the MLE of the model parameter * \(k\) is the number of parameters in the model
The model with the lowest AIC is the best model.
*
Bayesian Information Criterion (BIC): The BIC is another measure of the goodness of fit of a model. It is calculated as:
$$\text{BIC}= -2\ln(L(\hat{\theta}| x)) + k\ln(n)$$
where: * \(n\) is the sample size
The model with the lowest BIC is the best model.
*
Cross-validation: Cross-validation is a technique that can be used to estimate the predictive performance of a model. It involves splitting the data into a training set and a test set. The model is fit to the training set, and the predictive performance of the model is evaluated on the test set. The model with the best predictive performance is the best model.
Bayesian Inference
Bayesian inference is a type of statistical inference that is based on Bayes' theorem. Bayes' theorem is a mathematical formula that allows us to calculate the probability of a hypothesis given some data. It is written as:
$$P(\theta | x) = \frac{P(x | \theta)P(\theta)}{P(x)}$$
where:
* \(P(\theta | x)\) is the posterior probability of the hypothesis * \(P(x | \theta)\) is the likelihood function * \(P(\theta)\) is the prior probability of the hypothesis * \(P(x)\) is the marginal probability of the data
The posterior probability is the probability of the hypothesis given the data. The likelihood function is the probability of the data given the hypothesis. The prior probability is the probability of the hypothesis before any data is observed. The marginal probability of the data is the probability of the data, regardless of the hypothesis.
Bayesian inference can be used to make inferences about the model parameters. The posterior distribution of the model parameters is the distribution of the model parameters given the data. The posterior distribution can be used to calculate the mean, variance, and other properties of the model parameters.
Statistical modelling and inference using likelihood is a powerful tool for understanding data. It can be used to make predictions about the population from which the data was drawn, to test hypotheses about the population, and to select the best model from a set of candidate models. Bayesian inference is a type of statistical inference that is based on Bayes' theorem. It can be used to make inferences about the model parameters using the posterior distribution of the model parameters.
4.6 out of 5
Language | : | English |
File size | : | 31501 KB |
Text-to-Speech | : | Enabled |
Screen Reader | : | Supported |
Enhanced typesetting | : | Enabled |
Print length | : | 543 pages |
Lending | : | Enabled |
Do you want to contribute by writing guest posts on this blog?
Please contact us and send us a resume of previous articles that you have written.
Best Book Source
Ebook Universe
Read Ebook Now
Digital Book Hub
Ebooks Online Stores
Fiction
Non Fiction
Romance
Mystery
Thriller
SciFi
Fantasy
Horror
Biography
Selfhelp
Business
History
Classics
Poetry
Childrens
Young Adult
Educational
Cooking
Travel
Lifestyle
Spirituality
Health
Fitness
Technology
Science
Arts
Crafts
DIY
Gardening
Petcare
Alfred P Sloan Jr
Jorge I Klainman
Christine Schimpf
Allen Johnson
Jeffrey Goldberg
Ruthe Winegarten
Harvard Business Review
Benjamin B Olshin
Inge Sargent
Dorothy Tannahill Moran
Ellie Midwood
Robin Renwick
Jannie Geldenhuys
Christopher F Baum
Gina Pink
Nick Bilton
Tilman Borgers
Frances Osborne
Amy Bucher
John K Stutterheim
Light bulbAdvertise smarter! Our strategic ad space ensures maximum exposure. Reserve your spot today!
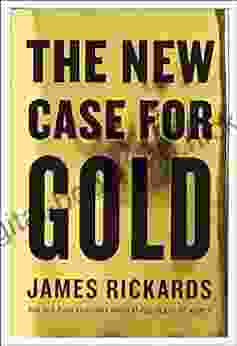

- Shaun NelsonFollow ·15.5k
- Anton FosterFollow ·4.4k
- Ted SimmonsFollow ·16.5k
- Richard AdamsFollow ·10.4k
- Gil TurnerFollow ·5.1k
- Jake CarterFollow ·17.9k
- Langston HughesFollow ·19.8k
- Ralph TurnerFollow ·12.4k
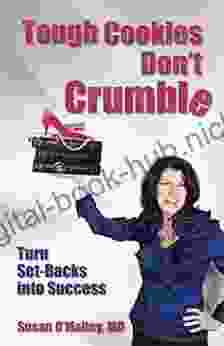

Tough Cookies Don't Crumble: The Unbreakable Spirit of...
Life is full of challenges. We all...
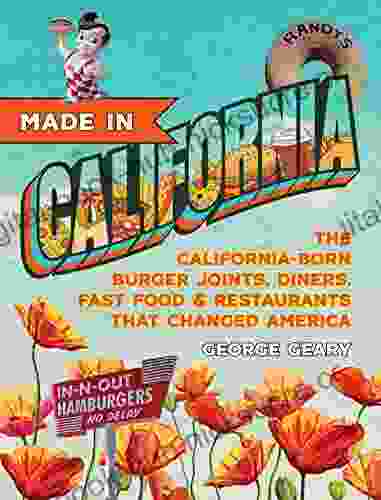

The California-Born Diners, Burger Joints, and Fast Food...
California is known for...
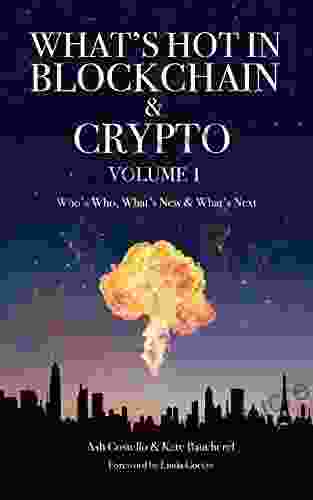

What's Hot in Blockchain and Crypto Volume
The blockchain and...
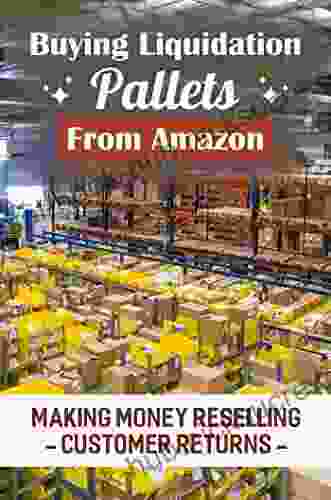

The Ultimate Guide to Buying Liquidation Pallets from...
Buying liquidation...
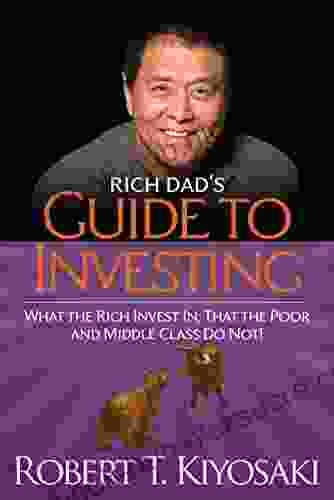

What the Rich Invest In That the Poor and the Middle...
The Secrets of Building True...
4.6 out of 5
Language | : | English |
File size | : | 31501 KB |
Text-to-Speech | : | Enabled |
Screen Reader | : | Supported |
Enhanced typesetting | : | Enabled |
Print length | : | 543 pages |
Lending | : | Enabled |